2018-09-05
A number of adversarial attacks on neural networks have been recently proposed. To counter these attacks, a number of authors have proposed a range of defenses. However, these defenses are often quickly broken by new and revised attacks. Given the lack of success at generating robust defenses, we are led to ask a fundamental question: Are adversarial attacks inevitable?
We identify a broad class of problems for which adversarial examples cannot be avoided. We also derive fundamental limits on the susceptibility of a classifier to adversarial attacks that depend on properties of the data distribution as well as the dimensionality of the dataset.
A full description of these results, and experiments exploring their implications, is available in our article.
Existence of adversarial examples
We assume that images have their pixels scaled between 0 and 1. We also assume that images in an object class are sampled from a probability density function with maximum density \(U_c\). Under this assumption, we can derive the following fundamental limit of the susceptibility of a classifier to adversarial examples.
Sample an image \(x\) at random from the class distribution. Choose any measurable classifier on the space of images. Then, with probability at least
\\( 1-U_c \exp(-\pi \epsilon^2)/(2\pi) \\) one of the following conditions holds true: 1) The point \\(x\\) is mislcassified by the classifier, or 2) There is an adversarial examples, \\(\hat x\\), in a different class, but with \\(||x-\hat x||_2 < \epsilon\\).
This bound guarantees the existence of adversarial examples if the density bound \(U_c\) isn’t too big. We provide theoretical results to show that, for very simple image classes, \(U_c\) gets big as the dimension of the problem increases. However, we also provide experimental results suggesting that complex image classes may have much lower values of \(U_c\), which could result in a highly non-trivial susceptibility bound.
Sparse adversarial examples
We consider the case of sparse adversarial examples, where only a subset of image pixels are manipulated. In this situation, we can prove that adversarial perturbation is possible using a small subset of pixels, provided the density bound \(U_c\) doesn’t grow too large.
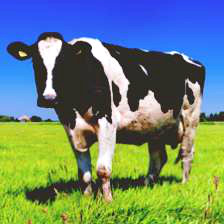
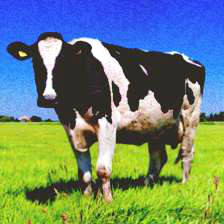
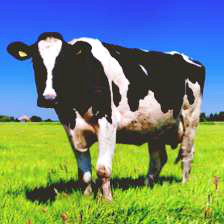
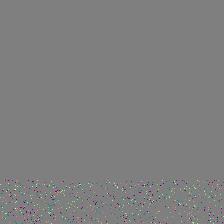
Sparse adversarial examples perturb a small subset of pixels and can hide adversarial "fuzz" inside high-frequency image regions. The original image (left) is classified as an "ox." Under \( \ell_\infty \)-norm perturbations (center-left), it is classified as "traffic light," but the perturbations visibly distort smooth regions of the image (the sky). These effects are hidden in the grass (center right) using sparse perturbations limited to a small subset of pixels (right).