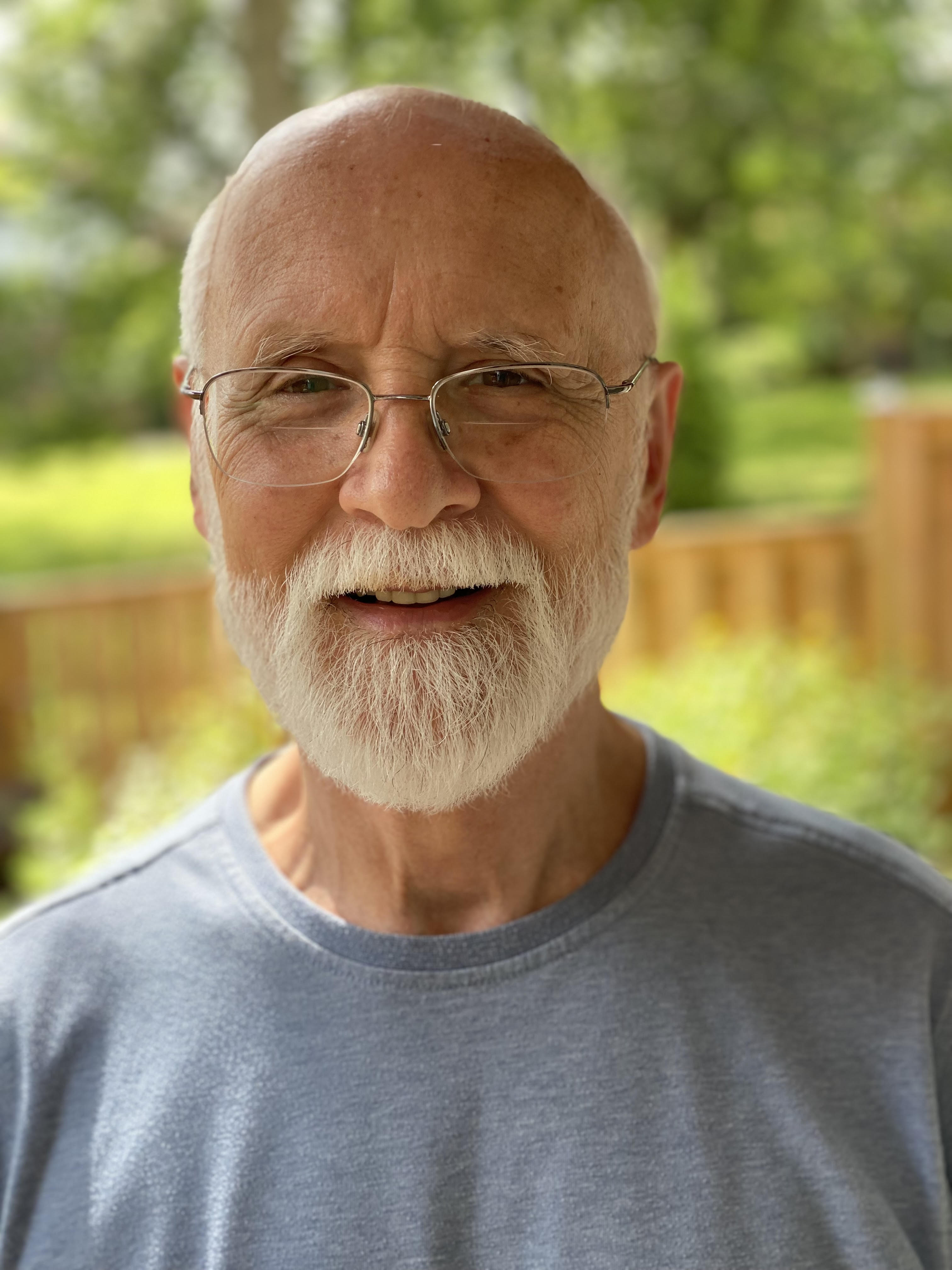
James A. Reggia
Research Interests
Research Interests and Selected Publications
Some Recently Taught Courses
CMSC 289I: Rise of the Machines - Fall 2016
CMSC 389F: Reinforcement Learning (advisor) - Fall 2018
CMSC 421: Introduction to Artificial Intelligence - Fall 2021
CMSC 422: Introduction to Machine Learning - Spring 2020
CMSC 727: Neural Computation - Spring 2022
CMSC 828Q: Nature-Inspired Computing - Fall 2019
 
 
supplement
CSD97